Season 1 · Episode 10
Data Analytics: Hard Skills vs Soft Skills and the Gift of Thinking Different with Karen Jean-Francois
Episode Guest
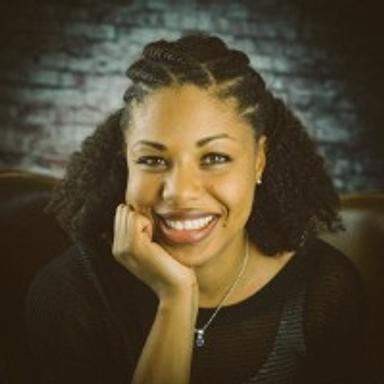
Karen JEAN-FRANCOIS
Senior Data Analyst at Cardlytics, Host & Producer of Women in Data Podcast
Senior Data Analyst at Cardlytics, Host & Producer of Women in Data Podcast