Season 2 · Episode 7
Data Management Pain Points and Future Solutions for Data Discovery
Data discovery is one of the hardest problems to solve in data management in general and comes up as a major pain point in most data mesh discussions. Tune in to this all-star expert panel recorded in collaboration with the Data Mesh community, and hosted by a previous Open||Source||Data podcast guest, Paco Nathan of Derwen.ai. Paco engages panelists, Shinji Kim (Select Star), Sophie Watson (Red Hat), Mark Grover (Stemma), and Shirshanka Das (Acryl Data) in a 60-minute discussion on not only Data Mesh, but other data strategies and process needs for the data discovery future.
Episode Guest
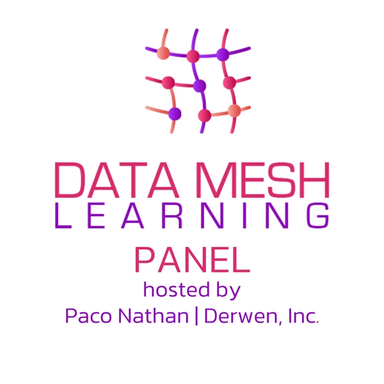
Episode Transcript
Paco Nathan:
All right, welcome. My name is Paco Nathan, and I'll be the host. We have through the data mesh learning community, and also in cooperation with one of my favorite podcasts, Open-Source Data. Folks on both teams have kindly been working together to put together this panel discussion. And we're here today to talk about data discovery in the context of data mesh. I'll just kind of frame it a bit first off by saying that there definitely have been some fantastic discussions about what is data mesh and how can you start to use this as an organization. This panel is looking more at the other issues that are surrounding data mesh that are very tightly coupled. So we're going to talk about data discovery here vis a vis data mesh and also a lot of other kinds of practices that are tangential and very much involved in these kinds of practice.
Our overall goal for this is we're going to throw some hard problems at expert solution architects and really talk through where do we have common ground? What are the issues we agree on or disagree? Not get into too much in the weeds about data mesh, but look at a lot of the important issues around it. And really, what are the practices that are needed going forward for data discovery? So collectively folks here have an amazing amount of hands-on experience and it ranges across a number of areas, data engineering, data science, data management, ML Ops compliance, customer solutions in general. So I'm really super thrilled about our speakers and what kind of background they really have to share here.
First off, I'd like to introduce Shinji Kim. Shinji is founder and CEO of Select Star, an intelligent data discovery platform. It automatically analyzes and documents your data. I'm especially impressed, Shinji, your background, you sought very challenging roles over the years and developed amazing background of complimentary areas. I loved the podcast interview that you do with Sam Ramji recently and talking about how a lot of that kind of background has come to bear in data discovery, which I feel is a very interdisciplinary kind of practice in essence. Welcome, Shinji. Thank you very much for joining us here.
Shinji Kim:
Thanks, Paco. Excited to be here. Yeah. Having spent a lot of time as a software developer, data scientist, data analysts, product manager in the higher tier, I think data discovery is something that affects all of us, whether you are on the full user or consumer side. So excited to talk about that, with also other amazing panelists here that have spent a long time in data.
Paco Nathan:
Fantastic. And next up, Mark Grover. Mark is founder of Stemma and co-creator of an open-source project called Amundsen. Mark and I have been collaborating on different things since the days when big data was young, back in the early 2010s and definitely throughout your work at Cloudera and Lyft and other projects that we've worked together on. Certainly you and your team has some of the most popular tutorials back in your early conferences when we talked about big data solutions. Welcome, Mark.
Mark Grover:
Thank you, Paco. It's great to be here. Appreciate you having me. And like you mentioned, we started big data when we were little kids. So we've been doing this for a while and yeah, I've had the good fortune of working with Paco in the past. And especially when I was Cloudera, where I was an engineer and I ended up co-authoring a book called Hadoop Application Architectures that sold over 10,000 copies. That put us in touch.
Since then I've gone from engineer to a product manager and created Amundsen at Lyft and excited to be a part of the discussion today with these amazing panelists.
Paco Nathan:
Fantastic, wonderful. Sophie, welcome. Sophie Watson is principal data scientist at Red Hat and, Sophie, I've got to say we've done a lot of speaking together in similar conferences and tracks. One of the things that strikes me is consistently when a lot of experts are up at the stage trying to explain the nuances of complex practices that are emerging, I found that you always come away with the best illustration that the audience can really understand the topic finally. So I'm super impressed with your background in data science and data engineering and really being able to convey and connect with the audience about these kinds of complex topics.
Sophie Watson:
Thanks, Paco. That's really kind. I learned everything I know about conference talks and illustrations and communication from William Benton. So I cannot not give Will a shout out on this panel. Access to data is a hurdle that every data scientist has hit at some point in their day, probably already, it's only 11:00 AM here, but I think we've all been bitten by this. So obviously, I want to be part of this community and learn about how we can make these problems go away. So thanks for having me.
Paco Nathan:
And Shirshanka, welcome. I'd like to introduce Shirshanka Das who is the founder of LinkedIn Data Hub projects, a very popular project in the metadata management space, also Apache Goblin, and now founder of Acryl Data. Shirshanka is also a co-author of a very seminal paper, it's called Ground: A Data Context Service. This is a paper, I believe it's 2017, but it really framed a lot of this general area of problems that we're working with.
And also, Shirshanka, we've collaborated on the metadata day events. And you've done a couple of those. I'm super impressed with how you've helped build community and dialogue around these topics.
Shirshanka Das:
Thanks, Paco. It's been great collaborating with you in the past. I'm so excited to be here and talk about this topic. It feels like data discovery is like a new term that we're talking about these days. And what I was reflecting on kind of the last 10 years working on data at LinkedIn, building online storage systems, streaming infrastructure, and then big data systems. This problem has been there at the center all the time. Like how do we make data easy to access? How do we make consumers understand what data they are really using? And I think most importantly, and this is why I'm so excited about data mesh, how do we make producers give them the mechanisms they need to produce high quality data products?
So I'm really excited to be here and be collaborated with Joe on the ground project that kind of laid some theoretical underpinnings I think inspired a lot of projects. In fact, when we built a third generation of metadata at LinkedIn, a lot of those things were influential in how we designed Data Hub. And I'm really humbled by how it does enable data discovery for so many organizations around the world. Super excited to be here.
Paco Nathan:
Well, let's jump into some discussion. Thank you all. So first off, let's get this started. Why is data discovery important? And actually, I'll throw this question to you, Shinji. Can you frame, why is it that data discovery is its own area that we should be looking at?
Shinji Kim:
Yeah. So the way I think about it is that overall in order for you to utilize your data or analyze your data... You're collecting data in the first place because you're going to use it somewhere. We see this as more of a pattern where you first need to have data access. You need to be able to touch the data, but then once you have the ability to access the data, you do need to understand what exists and where it is in order for you to start query, analyzing or actually making any decisions based on that data. And that part is what I would call data discovery, being able to find and understanding the data, which is, I would say, is in the critical path of any data analysis, any modeling or any manipulation of data.
So that's why I would say it's very fundamental thing that's important to just overall, for data professionals and industry.
Paco Nathan:
This is also, to characterize this, this is a very dynamic kind of area. It isn't like you just set a catalog of schema and it's written once and then used many times. This is something that's changing all the time, right?
Shinji Kim:
Yeah. I would say what's changing more now is with the rise of modern data stats, more companies being able to collect data, not just from their apps and websites, but from many different stats tools that they use and being able to connect those data with product data in one place is definitely... The area of data discovery, I think, is very much being looked at again by a lot of other companies, because now it's not also just the centralized data team that's using the data. There are product managers, often analysts, sales, ops people and engineers that are continuously looking at and finding different parts of the data that the company owns.
Mark Grover:
And if I may add on, there's another interesting thing here, which is why now is the right time to solve this problem. And I think Shinji explained really well, what is the problem and what it means and why it's important. But I find that over the last five, ten years, we've done a lot of innovation in getting data into a centralized place. So we've got like Stitch, Five Fan, a whole bunch of other systems, Kafka, that let us bring data into the organization. Then we've invested energy and infrastructure like Snowflake, Big Query are a huge thing now, which allow you to centrally store this data. And then we've had tools like Tableau, Looker, Mode that allow you to do analytics. There's people we have democratized who have access to data to these people. And so they're hiring data-driven product managers, data-driven sales enablement people. So the organization, A, has people hungry for data. B, has tons of data.
So the problem isn't that we aren't bringing data in or that the tools to consume data aren't there. The problem is there's so much data that no one has any idea what data exists, all these things that Shinji mentioned, what data exists, why it exists, how it's being used, where is it, can I trust it, all these things are the things that are preventing data from being used in the organization. And that's why this problem is so important to solve now.
Shinji Kim:
Yeah. I'm glad that you bring that up, Mark, actually, because I think it's so true for a lot of organizations today, primarily because you are moving your centralized data warehouse into the cloud. And the way that we are now ingesting and processing data is not through the ETL phenomenon. It's now ELT and with the transformation that all happens on top of the data lake or data warehouse, you actually end up with more data sets. That's confusing to not just the data team, for everyone else. But the phenomenon around decentralized ownership and maintenance of datasets under that data lake or data warehouse as well as a lot more distribution of data access by, not just the data team, but also everyone else inside the company makes data discovery a problem or a issue for a lot wider audience. And I think that's why, it's the why now is this a really important thing. It makes sense more.
Shirshanka Das:
I think another, if I can add one more thing that's happening is that even our conceptualization of what is data has changed. Initially, it was just tables in the warehouse, but nowadays it's much more than that. It's yes, the BI dashboards, the reports, it's also the ML models and the features and that's on the consumption side. But then on the production side, we're starting to talk about the Kafka streams upstream of the warehouse. We're starting to talk about the post-grads and the operational databases upstream of that. And then we're also starting to talk about the APIs that are kind of the front office for the company, and really trying to get an end to end understanding of what is the flow of data from the time it enters the organizations, kind of data boundaries, to the time it leaves it and everything that touches it needs to be understood and mapped.
And what I see repeatedly in the community, both in our open-source community, as well as in customers and the industry at large is that they like too many tools. And it's not just the data that is in different places, but it's just too many tools. This kind of hyper specialization that we're on has created a new problem of sorts that people just don't know where everything is at, and if I want to replace my BI tool with another thing, I just don't know what is going to get impacted and where everything is at. So I think that, and data mesh maybe is going to accelerate that a bit more as domains get even more autonomy to decide their whole stack. It's going to make it even harder and more important to understand where everything came from-
Harder and more important to understand where everything came from and where it's going.
Sophie Watson:
Right, and I think, we've got more and more data, like you said Shirshanka, it's been used all the way through the chain and people are thinking about it at every point, but that means more and more personas are interacting with it as well. So you have people interacting with data and making data-driven decisions, which is fantastic, and using all this information that's out there, but they don't necessarily have kind of a strict background in understanding data or doing this in a sensible and ethical way. So anything that can make that data easier to find, easier to understand and easier to use responsibly is a winner.
Paco Nathan:
Fantastic, I want to just catch briefly, there were a few terms that came out and for some of the folks who maybe haven't heard them before, one phrase was about ETL versus ELT and another phrase was about upstream as in like upstream Kafka. Could somebody take in break those down a bit?
Shirshanka Das:
We can talk quickly about ETL versus ELT. The old school philosophy was around transforming a lot of data in flight before loading it into your warehouse. Companies like Informatica pioneered the ETL movement. I still think they did a fantastic job at creating kind of that single holistic view of how you're modifying your data. But what has emerged over the last couple of years, I think, even when we were doing kind of our Kafka tail back at LinkedIn, I noticed that we were moving into this model of storage is cheap, data lakes are plentiful. Let's just copy all the data into the data lake or the warehouse, and then transform it later because the warehouses have evolved to handle that kind of abuse, for lack of a better word. And so don't throw away data in flight. You might need it. Just copy it all in from your source systems and then decide to transform. So that's how the ETL, ELT movement that has definitely happened.
It also leads to what I noticed simpler and more operable pipelines. I think I had a background as a platform engineer, so it's much easier to copy bytes over and to say, did I get it all, right? Versus I'm copying bytes and I'm also transforming and doing a few joins as I go and oops, I lost a bunch of data, but maybe that was intentional, maybe that was not. So that's definitely been one reason why it has caught on. It leads to more operable and simpler pipelines because cross system things are anyway hard, why do you want to introduce transformation in the middle of it?
Paco Nathan:
There's another term that definitely hear a lot about in terms of reverse ETL with respect to machine learning. Because a lot of what we do, uses of the data and data science teams, we might be doing annotations, or we might be doing some sort of embedding work with deep learning, but then we end up with additional products and it has to blend back in with our original data.
Mark Grover:
Yeah, but the guy with the chuckle I'll take the first best of this, but we'd love to hear other people's thoughts on this too. Yeah, it's that relatively new category. There are two products in this space that are often get talked about census and high touch. And the idea is that you can take the transform data that you have in your warehouse and stick this back into the systems that business users and [inaudible 00:18:25], right? So you have your customer success person who is always in Salesforce and they're using this thing to actually decide which customers do I support today based on some model that was run in my warehouse that produced my data and stuff, and so I think there's a big open question as to how are these reverse ETL tools different than the ETL tools that exist? And if they should be the same or different.
And I think there are different, varying schools of thought in here, but what is clear is that there's a deep need for taking the learnings that we have stored in the centralized warehouse and one place where people have done some learning and analysis and put them back in the hands and the flow of the users who are going to make that decision. And that is so such a core tenet of anything that we should be doing in the data space. It's like, how can you, especially with the fragmentation occurs, how can you take some insight that's buried somewhere and put that in the flow of the user, who's going to use that insight. And the flow of the user may actually mean that it has to go to a different tool than yours, and they don't have to come to your product in order to see that.
And then you push it out in their way. So they find it when they need it the most.
Paco Nathan:
Alrighty, let's go to some other questions here. Thank you very much for breaking that down. We've also had some questions coming in and I think we'll bring these up as well, a little bit more toward us, some strategies. So let's get through some of the more intro material here first. It's important, I mean, here we are in the data mesh learning community. Can we set the stage for what's the role of data discovery in data mesh and Shirshanka, you had started to discuss this some, can you discuss a little bit, go into a little bit more detail about that?
Shirshanka Das:
Sure. I think, the data mesh is all about splitting up your centralized data ecosystem into data domains and then applying a really high quality data product, thinking to how data is shared across those domains.
And I really think about data discovery as the capability for enabling that metadata plane or the control plane on the data mesh, right? When you talk about service discovery and a lot of the data mesh terminology has been inspired by the service mesh terminology. So when you think about service discovery, well, what is service discovery? It's really, the pods or the services registering to, is Zookeeper still a thing these days? The Zookeeper or Etsy nowadays, I guess, and saying, here's where I'm at. And so how do we apply the same thing to data? Well, data products should be registering themselves. And I think one of the things like data discovery has initially attempted to is really do as much of scraping and understanding of log files and understanding of stuff on the ground to be able to stitch together somewhat of a picture of what you have.
But what I'm really excited about is with data mesh, you can kind of invert that model and say, we don't have to be defeatist anymore. And we can actually have data product push metadata out from the data domains into the central metadata fabric. And that leads to much higher quality data discovery experiences, because you're actually producing data products that have a much higher bar for existing. It's not just enough to have a name. You also need to have an owner and you need to have above this schema. And maybe you have your compliance tags attached with you as you produce yourself. I'm really excited about what data mesh can do to simplify a lot of the hill climb, being that data discovery practitioners have been doing over the years and trying to stitch together facts on the ground
Shinji Kim:
For data mesh, I think it's really interesting because like a lot of companies are basically realizing that they already had all these different domain experts creating their own models and different data sets inside the data warehouse or even just in different parts of the data system. It could be very different databases, but it's still existing inside the same company infrastructure, right? But I think the full notion of data mesh the way I think about it is it's really the extension of how you're now recognizing that yes, it like the way that we model consume produce data is in a way, oh sorry, all day coupled. So almost like how we are moving along, so from like ETL to ELT, which I feel like is very much of a decoupling of you just build the data first and then worry about the transformation after. Also, with data mesh, different domain owners that should own and manage their own models.
And I think with decoupling, the parts that are also really important is this aspect of, so if I want to access a data or something worth or the service that I didn't create, but want to utilize it, how am I going to find out or understand how to use it without having to build it again myself, right?
So I think that part of our data mesh is like where data discovery affect a lot. So some of the customers that we are working with right now today have this data mesh model already. Initially they will start utilizing select star as a data discovery platform, so that initially they are just like finding out about the data, which I felt like was like a very much of a ware discovery kind of can start from with data mesh. But then, as it, they are starting to put tagging, ownership and then starting to invite their different team members of the company, they can basically provide a way to also have some, I don't want to call it just full governance or control, but wait for maintaining a source of truth while the ownership is still decoupled. And I feel that's the beauty of data mesh and it can something that can work really well with a good data discovery. So that's kind of how I see the intersection of data discovery and why it's so important for data mesh.
Paco Nathan:
Really interesting, so I mean, when we hear the word governance, on the one hand, there's this idea of the Magna Carta and some sort of federal judiciary and, it's a really elaborate system, but what you just described was more matter of practice, within an organization, a lot of people in the organization who are touching the data, what are their governance practices and what you're describing there is, are ways of making it so they can actually be engaged in it. They can actually be [crosstalk 00:25:24]
Shinji Kim:
Yeah, and also visibility, just being aware of what it's actually created or what other team has already done, gives you a lot of context and you can, reuse it instead of building everything on your own.
Paco Nathan:
Mark, you had some experience on this I'll quote from, or paraphrase, rather from some of your articles about experiences at Lyft. But this was one of the learnings when you're developing Edmonson, if I recall. I think a lot of data teams in my experience, leading data teams, we spend a lot of time relearning the metadata over and over and looking it up. And as Shinji was describing, I know I have in the past, you leave one business unit for six months to work on different business units, problem that you come back to it, the metadata is all changed and you have to rediscover it all over again.
Mark Grover:
Yeah, absolutely. I think the thing that Lyft was, I had gotten there from, after working at Cloudera for five years, and most of Cloudera customers were very different nature than Lyft and Lyft was all on the cloud.
They were, they had this data warehouse and they were growing double every year, right? So we went from a thousand to 2000, 4,000 to 8,000. And the thing was, we were hiring all these skilled people who had the knowledge of making data driven decisions, but didn't have the context within the company. And I remember this data scientists, one of my first user interviews that left was a data scientist working on ETA, which a time a cart driver it takes to get to you, right? And so we've taken a Lyft ride to you, open your app. And I tell you like, "Hey, Sophie, your ride, your driver's two minutes away." Right? And you go through the funnel and this ETA keeps on increasing most three minutes, four minutes, your driver shows up at your door like seven minutes later. [crosstalk 00:27:12]
Sophie Watson:
Yes, I think we've all been there, right?
Mark Grover:
Yeah, exactly. So the problem [crosstalk 00:27:17].
Shirshanka Das:
Did not happen to you Mark?
Mark Grover:
Yeah, we have a much slower version of the app, [inaudible 00:27:22]. Now we get it.
Sophie Watson:
Mark can drive himself as well, that's why you are calling me out.
Mark Grover:
Yeah, so the problem with the ETA is that we measure ETAs five, 10 minute session. So even a simple question, like what was the ETA doesn't make sense because you have to ask yourself, well, when did you measure the ETA, right? And the other thing is we have all these models, logging data into the warehouse. Sometimes we would have shadow models. So you have the one model that's showing data to the user and other one running on the side that's never showing any data and it won't change any behavior, but it's being logged and you can't really tell which one was the right one in retrospect, right? But you also have like 20 models in the past that were in production at some point that never made it to production. So you look at your warehouse and you've got over 200 columns, so many dashboards that have something to do with ETA and simply asking the question, where is the source of truth data for actual ETA is in San Francisco over the last week was so hard, right?
And the canonical way to solve this problem historically has been, oh, we'll get a data steward. So it's either a full-time person or a volunteer responsibility. You assigned to somebody and be like, Shirshanka shall keep this particular tag as a source of truth and move it as my organization [inaudible 00:28:46], right?
The problem is in fast moving organizations, data is changing so rapidly that we can't keep this up to date. And there's no, people are busy. There's no formal data steward responsibility for them to keep this up, right? And that's the other reason why data discovery has become so important because curated means of cataloguing your data no longer work. And what you need is automation signals like this thing is last up this morning, right? This thing hasn't been updated for the last 10 months, this thing is powering X number of models or X number of dashboards, right? Your coworkers all use this thing. All these signals become super important in you informing what's trust worthy instead of relying on this curated information that someone is maintaining.
Shirshanka Das:
Oh, this discussion reminds me of another inconsistency that I had to deal with at LinkedIn when we were doing governance and governance often leads to compliance, are we allowed to use that word here?
Paco Nathan:
Yeah, exactly.
Shirshanka Das:
One of the things that happened was we had data hub and it was being used for kind of compliance tagging, and a few other things. And one of the things we often forget when we talk about data discovery and notice, in the last 30 minutes we haven't.
Forget when we talk about data discovery I notice in the last 30 minutes, we haven't talked about data discovery for code. Like most of the data discovery we talk about is always for humans. There's a person looking for a data asset. There's a person trying to understand. But really when you start implementing compliance, you can no longer have people going around and scrubbing data rows for GDPR compliance or things like that. That's probably an agent that's actually taking action on the metadata that you have. And so data discovery can not just be a human thing. It actually has to be consistent. When I search for page view event on data hub at LinkedIn, and I get this as the top rank results, and when [inaudible 00:30:44] wakes up at night and starts scrubbing page view event, it makes the data hub has to return exactly that same ID.
And so this problem has to get solved not only at the human level, but also at the code level. You need to have real programmatic abstractions where the data discovery abstractions for humans have to also apply to data discovery attractions for code. And we see this with feature registries and model registries and all of these things. These all need a backend that they can reliably ask at run time, I've got a model ID, where is it at? Give me the model. What are the compliance tags? What is the inference capabilities? What are the tags on this thing? So that I can at run time, apply the right policies before rendering this back. And I think that's kind of the next challenge for data discovery is to also apply to programmatic use cases.
Paco Nathan:
Well that ties into a question that's come up. And actually there's been a little bit of iteration on the question. I mean, we are talking about systems of people and machines working together. So we definitely have a lot of automation. We have a lot of code. We have models that are derived from data built through code, but there's also a lot of people. And if you were to draw a diagram about this, there's a lot of complex interactions throughout. One of the questions that's come up is this concept of info overload or I would probably break that down more into cognitive load. So yeah, I mean, we can have a company, gosh, the last several startups that I've looked at in Silicon Valley, one of the first things you do is sign up for about 20 different SAS services just to get your company going. I know I had to. And then you've got some metadata exhaust from each one of those. And you're trying to keep track of it which is, Shinji, what you were talking about earlier about all these different components.
So there's cognitive overload in both in terms of push and pull, which is what was being debated or discussed. It's possible that people could take the actions to go out and track down the metadata for every different system that they have to work with. And Mark, as you were saying, go back through and find out what is the ground truth. So I don't know which is the push and which is the pull. But does the onus fall on the person who's using the data to go out and do that due diligence? Or does the onus fall on, I think Mark you were describing earlier was about the people who are creating the data, the producers. What responsibilities do they have for making this discoverable? And what are some practical ways in the ground in working with customers? Where does that balance lie? Where does that trade-off lie? Am I making sense on this?
Sophie Watson:
I see the onus being on everyone. I see it kind of like open source communities, right? For new open source projects. People contribute in part time and love and effort in because they're getting something out of it eventually. I think if we are saying, okay, it's your specific role to go through and tag all this and catch all this information, then it's just not going to happen. I mean, that doesn't sound like that much fun to me if that's all you're doing all day every day. But when you see the benefits of actually using this as part of the wider community and see what it brings, then I think it gains the momentum. And so it's always hard to get started with something, right. But once you've kind of used this for a while, and then you find some data that doesn't have the information that you want with it, then I think you're more incentivized to go and add it there so that someone else doesn't have to relive the pain that you just lived. Mark?
Mark Grover:
Yeah, I totally agree with that. In fact, I just recently wrote a blog post in the two most common reasons why efforts around, I think data catalogs and data discovery or interrelated terms. And I wrote a blog post two most common reasons why data catalogs fail. And what Sophie described is actually the first reason, which is you don't have enough documentation in there in order to actually people to derive value. Right? And one thing that I believe strongly from having worked with many companies in the [inaudible 00:35:18] and open source community at Lyft, and now with customers Stema, is that the best time to get this documentation is when this is in the head of the person who has this documentation, right? So take an example, say you're creating a new event that's going to stream down to the data warehouse.
And this sometimes could be an event that's been created in the marketing, [inaudible 00:35:41] or segment or something like that. Right. Getting that information from them when they're creating that and enforcing checks and balancing right there, that if they didn't put that information that their code won't go through is like a great way to actually ensure that the description trickles down. Right. And then once it does trickle down and you're building derived sources, you can actually, a good chunk of the documentation can further trickle down based on the processing you're doing. Right. So, A, I'm saying that you can reduce the amount of documentation you need by understanding what is being built from what. But B, get the documentation in the flow of the user. And in my opinion, that would be the person producing the data in the first place. They have some context in their head and grab that from them right in that moment.
Shinji Kim:
I also want to add something to this. So yeah, I do also believe that having a good amount of documentation very important, especially for the [inaudible 00:36:38] side of the things. At the same time, there's so much data that also gets created or rephrased on top of the raw data and it just creates a lot more tables. So what do you do? Do you just copy and paste like well, it has already existed? That itself is a lot of manual work. And few things that we are starting to see that we feel like is the part that can be really helpful is kind of propagating some documentation that already exists from the upstream side to the downstream. But overall, I think regarding this overload of both producer side and consumer side. I think because I actually think on both sides actually have the responsibility of annotating or creating the data discovery platforms.
But I feel like for a lot of modern data discovery platforms, like the companies we are all working on, I also, just because I also wrote a blog post about this. How there's so much manual work that's not being automated. I think there used to be two main things that needs to happen. One is, automation. And then the second part is really easy user experience. So with the automation part, I'm talking about being able to pull out existing operational metadata to augment the discovery perspective so that the users can still find what they're looking for even if it may not not have a full documentation.
For instance, one of the things that we see a lot in Select Star is how people actually search a keyword and then being able to find the specific field of what they are looking for, even though the same exact field exists in the data warehouse. Same, thousands of other fields, like user ID exists in hundreds of other tables. But how do they know which exactly is their ID column that you are going to use? That will be driven by popularity.
And we calculate our popularity score based on the number of select quarries that mentions that ID and how often does it run, how many unique users are running. So those types of automated insights that people can use in order to foster this type of better documentation. When you know how the data is currently being used it is like a great ways to understand and start creating more documentation on top.
I think the other part that, both, I think Mark and Sophie alluded to, is putting in some process in place. So you [inaudible 00:39:18] process, you do have to add the descriptions per new column that you are creating. I think those are all really good best practices to put in. At the same, for the existing data, for all the a hundred thousand columns you have it maybe a little bit harder to enforce everyone to just do the documentation from the get-go. So I think having more of these automated insights, I think is one part that is very helpful and is something that a lot of companies are starting to support, including creating the lineage so they can propagate different information as well as the popularity and another part.
And then in terms of the user experience perspective, I think on the consumer side, how and where this data is being used? Is this data primarily used for sales report or products analytics? Things like that. I think it can also be very helpful to help others to recognize that these are the right set of datasets to use. And that itself can be also very much of an over load. So I think the other part where automation can help is was the data analyst team or more business intelligence teams can define the high level of a structure of a, how they would view categorizing the data.
Then other people can contribute on whether they, just by either taking the data. But the part that where automation can also help is by telling you, Paul is using these types of data the most of the time. And then by looking up which team they belong to, or which teams that use using which types of data the most, I think is also another piece that can really help the documentation or foster the initial documentation efforts that usually needs to also happen with data discovery. Sorry. I think I put up a lot of stuff out there. Yeah.
Paco Nathan:
Fantastic. [inaudible 00:41:18]. I want to try to paraphrase that a bit then also. So looking at data discovery and a lot of the issues and nuances here, Sophie, you were bringing up an analog with, say, open source culture and how people will find something that could be a lot better. Here's a PR let's fix this. If we find like here's something that we know is missing, let's go ahead and fix this. And there's, one of the things I love about working in open source is almost kind of a gamification of, like working through PRs and knocking down problems. And then Mark you're describing what I think might be an analogy of say, pre-commit hooks. You've got a project and you've got end developers working on a thing, and now let's go ahead and refine how we're using our pre-commit hooks so that we don't keep making the same mistakes over and over again, and try to catch it at closer to the source of the root cause.
And Shinji, you were talking a lot more about almost like a dependency graph like you would see in an open source packaging system. But a dependency graph for propagating metadata descriptions about data and data discovery. And then on top of that also, I think touching, you really articulated well about a lot of the kind of UX issues that come into discovery. Things have to be searchable. They have to be something that people can go in and find conveniently.
Sophie Watson:
Right. And searchable by everybody. We're not just talking about the people who understand what pre-commit hooks are.
Paco Nathan:
Right. Right. Exactly, yeah.
Sophie Watson:
We're talking about everybody who is touching this data needs to be able to confidently know why it is tagged in, have confidence in the tags that are there. And have the ability to contribute more to them without having to get account, in my opinion.
Shirshanka Das:
One thing that I've noticed resonate a lot in this is kind of tying into what Mark was talking about is kind of treating data as code and treating metadata also as code. And so essentially, we see it a lot in kind of the data mesh community, especially kind of the early adopters like Saxo Bank. LinkedIn was accidentally doing data mesh, we just didn't know that it was called data mesh back then. But having this ability to say, when you're creating a data product, a ping that has something more than just a schema associated with it, what are the rules for what makes it valid? And being able to apply those rules as part of the bail system, like it needs to have documentation on it. The compliance tags have to actually align with the glossary of compliance terms. That's actually a bill check. In the ID, you actually get auto-complete. So we need to have kind of the systems integrated into the individual tools so that the likelihood, just like with data, that we want the likelihood of producing that metadata to be as low as possible out of the gate.
And so your system, the data discovery tool or platform really has to support this kind of versioning of metadata and the ability to have lots and lots of versions of metadata attached to lots and lots of versions of code so that you get, [inaudible 00:44:34] to time travel through your metadata and time-travel through your code. And I think that really can unlock a lot of capabilities that we're kind of missing in our current tool stack.
Paco Nathan:
Well, there's a related question that came in and we've had wonderful questions coming in. There's a big stream. Probably more than we'll get to, but I'm going to try to weave them in. We've been weaving them through the conversation already. Noel O'Connor asked, does there need to be a promotion process? And I think, just talking to this goes back to something you were just saying, does there need to be a promotion process...
This goes back to some of what you were just saying. Does there need to be a promotion process to ensure that data is accurate enough and trustful enough, something akin to APIs and Kubernetes, where you have alpha beta GA?
Shirshanka Das:
Absolutely. Yeah, I think just like you can read services before you actually turn them on, you need to be able to kind of read data sets and you need to be able to say this data set is available, but its proof of life and its proof of goodness is not yet there because maybe the next person who tried to use it fails miserably. And that kind of puts it in a... and I see a lot of attempts at trying to give extreme versioning applied to the data ecosystem and I think it's a very exciting trend. And I think that can enable this kind of ability to achieve what we do with services, with data.
Paco Nathan:
Are those kinds of features available in, say, an open source, like that kind of promotion process?
Shirshanka Das:
Sort of. Sort of. I think we see capabilities where folks are checking in schemas with compliance rules. And then there are essentially GitHub actions that are able to check with the metadata service whether this particular commit is valid based on the rules that they have defined in the metadata service. And if it's not, then that data set can not be promoted. But I think the combination of metadata versioning and data versioning is kind of an evolving space. I see a few projects like NESI and [inaudible 00:46:39] coming up around kind of versioning of data assets. I'm curious to see whether that can be combined with versioning of metadata at kind of the logical level as we talked about as well. I think it's going to be pretty interesting.
Shinji Kim:
I think that notion applies really well on the data producer side and on the data consumer side I think the other notion that's also really helpful is utilizing tags. So for us, at Select Star, we have a notion of status ties and a lot of customers use it actually at least to define which are the thirty five datasets, so that new analysts or anyone that's not initially looking at the data like those with these are proven and tried and true. These are the analytics data tables that everyone is using, but also we've seen customers marking their tables as gold, silver, bronze, raw versus data. So yeah, alpha beta GA I think is definitely another way to utilize the type of tags and attached a certain status to the datasets on the consumer side of the things. And I think it needs to always happen on both sides, right? You can't do only one side, [inaudible 00:47:47].
Paco Nathan:
Always ensuring that the audit is both producer and consumer side. That seems to be a really key point here. I think some systems tend to bias one or the other.
Shirshanka Das:
Yeah. One of the things I have noticed with tagging is tagging itself is an interesting automation problem. Paco, I know you were kind of an expert on knowledge graphs, but even the fact that what makes a dataset a goal dataset might be something that someone stamps on a data set today and they had some policy in their head about what makes it a goal dataset, but they didn't encode the policy. Instead, they went and stamped the data set as gold, but next week that policy got violated, but the data set is still stamped as gold. And the policy maybe was, oh, this data set should land before 7:00 AM every day for the last week.
Maybe that was really the policy, maybe it's reliability policy that makes something a goal data set or not. But if we are able to actually encode those policies and say, if you meet those characteristics, you get the gold tag. Then that leads to a much more vibrant manageability of these tags themselves, where you don't have humans applying these tags, but the facts really are predicate on the metadata. And so you get the tag based on your performance, not because someone's stamped it on you. So that might be an interesting way to even manage these tags governance or bag application.
Shinji Kim:
Yeah, I think maintaining the status certainly can be done really well with having some workflow or automation built on top. For sure.
Sophie Watson:
I think my main concern with this notion of promoting datasets is exactly this, things getting tagged at time X and this just perpetuating, how badly problems perpetuate through machine learning systems because of X, Y, and Z.
And it's the same, but just with data, right? And the other thing is, I think in a lot of these cases, we're focusing on this notion of the same people, no, sorry, multiple groups of people using the same datasets in exactly the same way. So for exactly the same thing or to gain exactly the same insight, I want to push back from that a bit and say, if people are doing that, then you've got a bigger problem in your company. People are duplicating work somewhere. What we really want is the data to be used in new and interesting ways because it's easier to find and discover. And so I am interested to see how we progress over the next few months and years for tagging and boosting up datasets and stamping them as good.
Paco Nathan:
Well said. Sophie, what you're describing there really overlaps some of the current trends in AI.
And so shocking you used the word predicate. I think what we're talking about here is, this kind of metadata really does need to be inferred and inference is a hard problem. It's something where we can combine the domain experts and policy, but we can also combine the machine learning. And when you do have graph representations, then you can start to test the tags and the policies for things like uncertainty. So, looking at what Lisa and her team do at Santa Cruz with probalistic graphs, that would be a really ideal thing to try to apply, to figure out, Hey, wait, are the policies being invalidated by the tags that we've added or how much uncertainty does adding this next tag create.
Okay. I'll jump off that horse. I've been reminded we only have a few minutes left. Yeah. Okay. So there've been some questions definitely want to try to hit here.
Muhammad, you had some excellent questions. Mohammed Chopra asked at what limit does data discovery reach its limit when handling PII, personal identifiable information, and how can we try to consume this in a secure way? How can we make it consumable in a secure way? So, we've been talking about some policies for data discovery. What are some of the limits?
Mark Grover:
Yeah, that's a great question. And I think historically there's one approach here, which is if the data is not accessible to you say, because it's sensitive, that you should not be able to discover it. And I actually disagree with that approach and I've seen that not work for a good chunk of companies. And the reason I disagree with that is because it becomes a chicken and egg problem. If you don't know this thing exists, you don't know if you can use it for the work you're about to do, and therefore you can't get access to it.
So it prevents your decision-making ability using that data if you were, if you had a path to getting access to it. Right. And so the approach that I've seen work in modern organizations is that it's accessible, that such data exists, it's known that it's sensitive and then you don't see some richer metadata around it because of its sensitivity. So you may not see what Auris are being run on it. You don't see any profiling of the data. You can't preview the data, but the existence of the data is there for you, right? And it prevents the chicken and the egg problem. You can discover all the data that's present of the company. You know there's some sensitive information here, and then you can say okay, well this is what I need and I'll find a path to actually getting access to it.
And I've seen that to be a much more successful way. So to summarize that the path I'm seeing most companies adopt in my world is accessibility and understanding of data is democratized even for sensitive data, to a certain extent. And then richer metadata is blocked unless you have access to it. And when you get access to it, you can see that [inaudible 00:53:39].
Paco Nathan:
That's something that comes up in healthcare a lot is like de-identification is probably one of the top use cases for NLP now in AI, in healthcare. And so there can be a path even to sensitive data, if you can go through enough to de-identification.
Shirshanka Das:
Yeah, I believe strongly that metadata infrastructure should just mirror data infrastructure. So, just like in data we have our back and a back and the ability to anonymize data at point of access, we should have the same capabilities on metadata itself.
So if you have access to the data that implies, you have certain level of access to the metadata, and if you don't have access to the data that implies you have a different level of access on the metadata. We actually have companies that we are working with where even the table names are sensitive and a single domain does not even want another domain to know that this table name exists. So as we're building kind of the our back capabilities and the access control capabilities in data hub, we're having to design for those kinds of scenarios as well, where depending on the access privileges you have, you may not be able to even see the name of the table, but you might be able to see the tags on the table. So it's quite interesting. And I think as long as you mirror kind of capabilities that data systems have evolved over the years in terms of being able to support these kinds of graphs and find grain, access control capabilities, and anonymization capabilities, you can apply those same things to your metadata system as well and it should work, fingers crossed.
Shinji Kim:
And I think regarding the our back perspective, metadata systems, one of those things that we will be working on a lot of metadata systems, I think should also consider is any grand stands with the existing, our back policies. So you are either replicating the same roles to the users that have access to the data discovery platform, but they have exactly the same viewpoints from snowflake or big query, like what they already had, or the other type of, our back that I see, because we thought of our users are also on the [inaudible 00:55:49] or PM or work just the pure consumer side is actually doing the Arbonne replication where sync with the BI tools, because the AI tools may have a lot of sensitive data from that side.
I think the other part around PII is so as a metadata platform, we won't be accessing the data. But for us at, by default, we do access the query history and we will get exposed to whether we intend to, or not from somebody's query, if they are accessing a specific column, that may be a sensitive column. And for us, what we do today is that we allow customers to define, which are the columns that you put a tag on those columns. And we will remove those data in memory before it gets a bit into disk. So that anything that you access within a select star, even though you might have stepped into a query or anything, it's already masked out and anything that [inaudible 00:56:50] on top of it, he's already masked.
But overall, the definition of that PI, which are the fields that are sensitive, I think that is also a very interesting area that's more on data warehouses are starting to provide capabilities within because they already host the data. And I think companies like [inaudible 00:57:12] they already are starting to work on pointing out, which are potentially the sensitive data and allow customers to automatically tag them. So I think integration with that tagging, I think is also very interesting that can be replicated into the discovery system.
Paco Nathan:
We have a lot more, obviously a lot of interest here and we have definitely a lot more resources on the data mesh learning community, especially on the slack board. There's a lot of channels. And I know a lot of us are involved there. A lot of other experts too. So what I'd recommend is there's some great questions outstanding, but let's move it over to slack. And we can go into this in a lot more detail. And also we can dig into deep dives on some of the other questions that are come up earlier. Some of the other parts of discussion. With that, I want to thank everybody. So Pete, Mark, [inaudible 00:58:05] also, Scott for putting this together. Audra, I know you're back there. Thank you all very much and really appreciate the discussion today. There will be a recording of this going up I believe on YouTube and that'll be open to the public.
Thank you very much for your time and your insights today.