The Challenges of Current AI Architectures
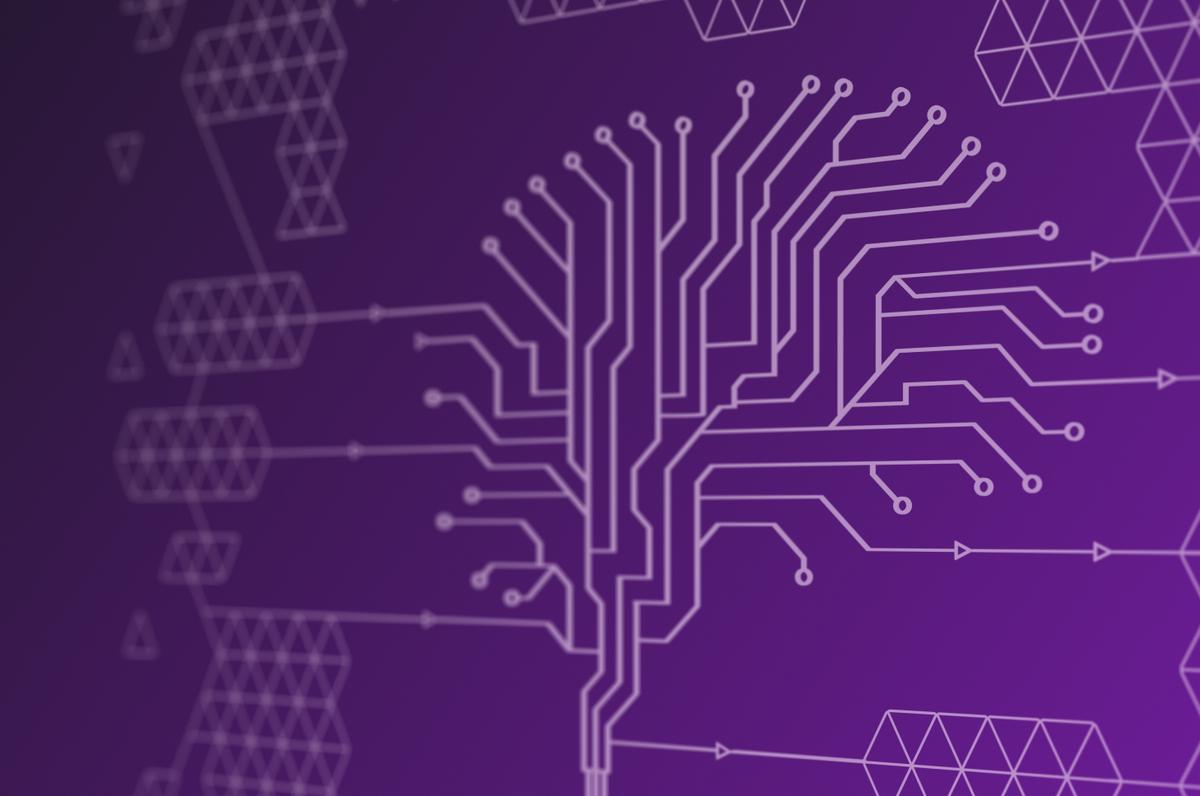
We’ve all seen the predictions. AI will be the most transformative technology of our generation. Nearly every business leader believes that AI is critical to success. The list of enthusiastic predictions goes on. But for all the hype and enthusiasm, reality has been much more challenging. A report by Accenture drives this point home by showing only 12% of AI initiatives succeed in achieving superior growth and business transformation. So why after years of investment and innovation do AI initiatives still fall short?
Here, we’ll delve into the four most common limitations present in AI and ML initiatives today.
A promise unfulfilled
Simply put, the process to build and utilize machine learning architecture doesn’t move at the speed of business. In fact, data scientists and developers are attempting to build the most powerful, sophisticated applications for the next generation of business on an infrastructure and architecture built for the demands of the last generation.
Compounding this, consumer expectations and business models built around digital experiences continue to evolve every day. Our choices are more varied, our engagement is more dynamic, and both consumers and businesses have more options than ever before. We have grown to expect that every digital experience is hyper-personalized and delivered instantaneously. This means that businesses must respond by capturing consumer opportunities the moment they happen. We all expect to be constantly engaged with a service from the minute we begin to the moment it is complete. Users order food for delivery the moment they need it. Transportation is expected to be available anywhere and everywhere and when we want it. The businesses that have already evolved to these real-time demands are the unique few that continue to thrive, even in today’s rapidly changing marketplace.
Artificial Intelligence is the type of technology that should produce instant, real-time insights that deliver on all these consumer expectations and business needs. Machine-to-machine communication promises to extract insights at a pace far beyond the speed of humans, with a degree of accuracy that exceeds human ability and from a volume of data that would overwhelm even the largest analytics teams. But ironically, it has failed to do so. The way these systems have been architected has created natural limitations to the quality and the impact that AI can deliver, resulting in predictions that fall far short of expectations, or are delivered too late to make a real impact in the business.
Demographic machine learning versus behavioral
One of the core limitations of ML/AI today is that it’s built primarily to predict individual behavior based on broad demographic data. This approach made sense at a time when serving real-time applications and real-time ML wasn’t possible and directional forecasting was considered optimal. Giving broad insights into users based on similar demographics over long periods of time was good enough to achieve KPIs or revenue requirements. However, this old approach has obvious limitations today.
Too many businesses are missing opportunities to tailor an experience or an engagement to the exact needs of an individual given their intent and context—independent of the broader demographics. Or they might mistake a single behavior as part of a pattern. This means missing the opportunity to adapt and prioritize an offer or an insight captured during a single session that might be more valuable than the entirety of the customer's engagement or pattern of behaviors. For example, to achieve a more impactful personalized experience, an online retailer needs to know the difference between a customer buying a baby shower gift, executing a one-time transaction, and a customer who's pregnant and entering a new phase of engagement with that retailer.
Batch processing in a real-time world
Further limiting the impact of AI is the type of data that it analyzes. The majority of AI systems were built around batch processing and historical analysis. On a nightly, weekly, or event-based schedule, data is collected from batch processing, which creates pre-aggregated tables with data from a warehouse, a file, or a bunch of other sources. However, because it has been scoped down to the smallest possible set of data in order to transfer the data to the ML, capturing real-time insights becomes prohibitively manual and complex.
Markets today move much more rapidly. Consumers have more options than ever and more opportunities to source products and services from a range of suppliers ready to serve them with the best product at the right price in the shortest time. This creates a dynamic environment that requires real-time inputs to get optimal results. For example, historical analysis may predict fluctuations in seasonal inventory, but fail at identifying unpredictable supply chain disruptions or variations in costs (such as fuel, shipping, and travel) that happen multiple times a day. Understanding data in real-time isn’t just about keeping customers engaged, it’s also vital to preserving margins, even as market conditions remain volatile. In the end, this outdated process of bringing your data to your ML architecture costs enterprises real-time insights and opportunities to meet the demands of today’s marketplace.
Time, cost, and complexity of bringing data to machine learning
The majority of ML systems are built around the basic concept of bringing data to the machine learning platform, to achieve directional forecasting. Even when real-time data is available, it’s analyzed through the same, often disconnected, process that is used to analyze historical data. This means that organizations will dedicate massive resources, time, and budget to migrate data from data warehouses and data lakes to dedicated machine learning platforms before analyzing for key insights. Not only does this result in massive data transfer costs, but the required time to migrate, analyze, and migrate again impacts how quickly we can learn new patterns and take action with customers in the moment.
Even when teams make course corrections, achieve alignment, and arrive at a successful outcome, the terms of that success often no longer apply. This happens through a myriad of factors, but obvious instances are the data is out of date, or the model is out of date, or the examples weren’t trained for the moment needed (like time of purchase, or time to select a movie, for example). All of this results in increased time, costs, underperforming models, or models that don’t work at all.
Siloed practitioners and tools
Anyone engaged in building ML applications—data engineers, data scientists and developers—often work in silos with very different goals. Often wide visibility or deep understanding of a ML project is out of reach to team members spanning the data, ML, and application stack. Data models are built to serve the applications and use cases as they exist today, not the potential ML models they may serve tomorrow. Confidence is difficult to achieve when bringing data to ML models unless you know for sure that 1) the data is up to date and will be available in production and 2) you understand that the transformations took place before taking a dependency; this introduces the risk that someone along the way changed (even slightly) the pipeline definition in the production environment or will do so in the future— making the ML model predictions wrong.
Additionally, the stack of tools utilized vary widely based on your place in the stack from engineering to data science or developer specializations. If one uses SCALA for building pipelines and another uses a streaming service to power their application while another employs some different service for building training sets, then chaos ensues because choosing these tools often has tradeoffs, and they’re often pointed at different data sources and sinks. Making necessary corrections can cause delays anywhere from months to quarters and even years, and not just one time, but everytime this process repeats itself.
Bring your AI/ML to the data
These problems are ubiquitous and create chaos for everyone involved and frustrations for executive sponsors counting on the impact of AI initiatives. These compounding problems create delays and, despite millions of dollars invested, ML/AI projects only achieve a minimal impact, far short of the promise and vision to deliver value, or they are simply deemed esoteric and banished to the AI project graveyard.
There is a better way to deliver real-time impact and drive value by using the power of real-time AI. In our next entry, we’ll explore how you can transcend these limitations and join the ranks of businesses that are bringing their ML to the data to deliver more intelligent applications, with more accurate AI predictions at the exact time to make the biggest business impact.
In the meantime, I encourage you to learn more about real-time AI from DataStax on our Real-Time AI page.
1 2022 Deloitte survey;
https://www2.deloitte.com/us/en/pages/consulting/articles/state-of-ai-2022.html