How Dataworkz Democratizes Access to AI and Data Analytics
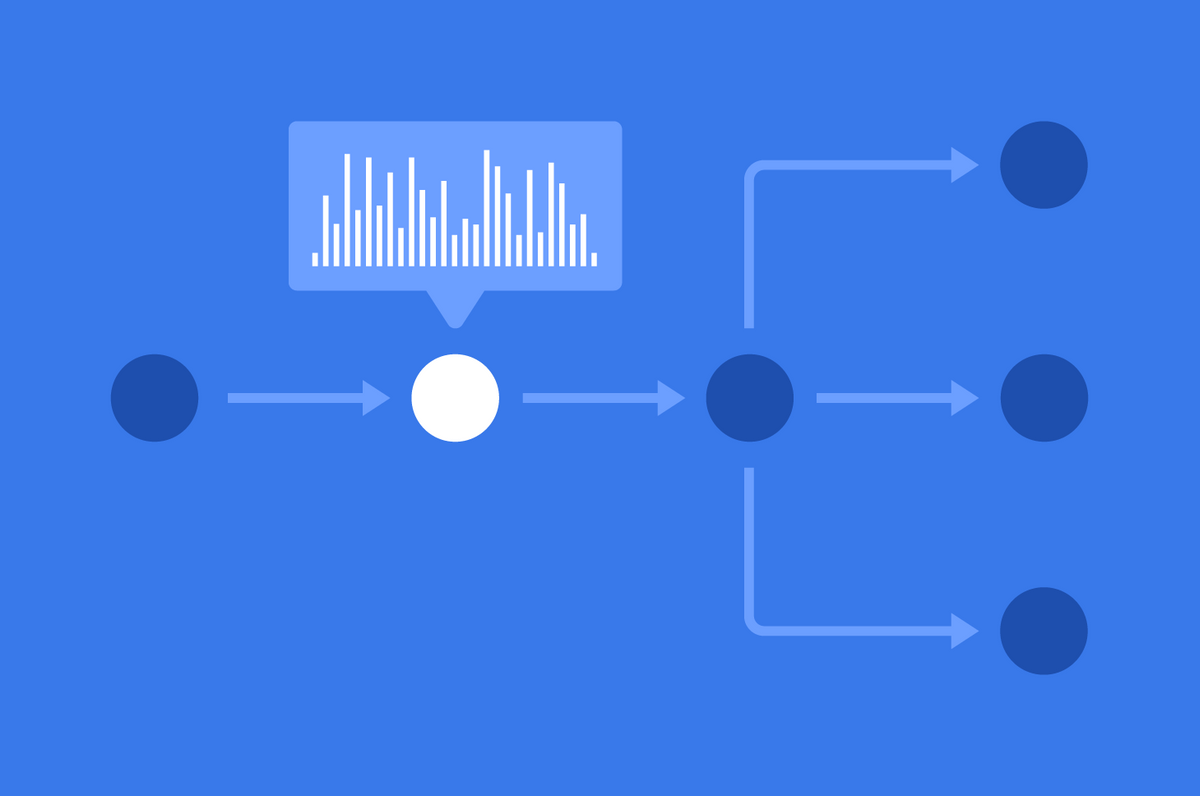
The complexities of analytics based on artificial intelligence (AI) aren’t always easy to master for the average business user. But Milpitas, Calif.-based Dataworkz is trying to change that by offering an easy-to-understand user interface for its no-code, high-performance cloud service that unifies data, transformations, and AI for business users.
We recently spoke with Nikhil Smotra, Co-Founder and CTO of Dataworkz, to find out more about the company’s mission, how Dataworkz uses Apache Cassandra®, and why the company migrated to DataStax Astra DB for a Cassandra database-as-a-service.
Tell us about Dataworkz
At Dataworkz, our core mission is to simplify AI-driven decision making. Our platform is offered as a service to enable creation of AI-based applications.
At founding, we looked at the entire landscape of machine learning challenges. In the first category, there is the deep tech which is completely focused on building new machine learning (ML) algorithms. A second category revolves around applying well-defined ML algorithms to the world of big data, wherein data is flowing in at a very high volume, velocity, and variety, of late.
There is growing interest in veracity, that is, tracing the data's journey from the source to the destination, and all the different steps which have been taken to transform that data during its lifetime. This is important because when people talk about building AI-driven applications and using ML models, your output is only as good or as bad as the data you're feeding in.
To prepare for ML initiatives, organizations are dependent on availability of data to run the algorithms against. Many times, companies have to use a plethora of technologies and tools which solve very specific problems, then stitch them together to generate the data sets. As a result, bringing in data and combining it all, at scale, has become much like a software integration problem, wherein you have a lot of handoffs between multiple teams and applications.
This means spending a lot of extra money as well as a lot of time, which could have been spent generating insights. That is where Dataworkz comes into play. We are unifying the data processing and AI into a single experience and offering that as a service. This allows business end users to go from knowing nothing about the data to understanding what the data means and to being able to transform the data. Users can run ML algorithms on the data and write it back to the operational systems in one single experience, instead of trying to use five or six different tools and then stitching everything together.
With this opportunity in mind, Dataworkz was founded in February 2020 with a seed investment led by Engineering Capital. Dataworkz has multiple production deployments with customers in high tech, legal services, life science, and more.
What challenges does Dataworkz help enterprises solve?
Our initial focus is on sales and marketing applications. For example, these days many new technologies are offered in a SaaS model, with free trials to incentivize signups. Companies try to make signing up as frictionless as possible, so they require only very basic details, such as an email address. And that’s it.
A company signing up thousands of trial customers every day needs to figure out which customers are going to convert into paying accounts. In addition, to improve prediction rates, companies would like to map the trial data back to enterprise account records in Salesforce and other CRM tools, but the machine learning algorithms which come out of the box are insufficient.
Dataworkz solves the challenges of matching trial accounts with the enterprise accounts when there is no common identifier between them. This can be thorny, whether you have a user’s personal or corporate email address. There can be questions around which similarly named enterprise account record in the CRM corresponds to the trial user or office location. You might need to map to IP addresses and geolocation information.
A complicated way to handle this requires a series of steps, starting with buying an ETL tool to extract all the CRM data and put it into a cloud data warehouse. Because there is no matching key between the trial accounts and the enterprise accounts, you would have to use some fuzzy matching algorithms. Then you would need a reverse ETL tool to update this information into the CRM system, where you might run into issues with subsets and children of parent enterprise account records. The whole process requires multiple tools and is very time-consuming.
Plus, you have to hire data engineers with specialized skill sets to look at the data in the cloud data warehouse and do all that work to enrich it. It also requires the sales and marketing organization to be very dependent on IT. Many times, the IT team is extremely busy and can only get to this type of request based on its priority queues. Then, by the time they get to the request, say three months down the line, the business requirements have changed because today things are moving at such a fast pace.
The alternative approach is to use a platform like Dataworkz, which can solve all these problems in a span of 10 to 15 minutes—instead of weeks and months—and within a unified experience. It’s a use case where we really shine. We’re in production with it for several customers.
What is your perspective on collaboration between data consumers and data producers?
One of the key features we wanted to base our product on is the collaboration between data consumers and data producers. That is the most important aspect, which not too many people have even thought about in the data management space.
Consider the situation of a marketing ops team building a funnel for a marketing initiative, when someone from the sales organization decides to delete or add multiple fields to the same CRM objects. How do the right people get notified about this change? How do you make sure that whatever changes the sales team makes don’t affect the work of the marketing team?
Why did Dataworkz become a DataStax Astra DB customer?
Dataworkz builds collaboration between consumers and producers using the concept of an activity stream. The activity stream is also used to learn user behavior and improve product experience over time. We wanted to build a solution that was really fast and was easy to set up and maintain. It needed to be set up so that as the load on the system increases, it automatically scales out or scales back in a cost-effective way.
My team had a couple of experienced Apache Cassandra developers and we needed a columnar store with the benefits of compression so we decided to choose Cassandra. To track a high volume of activity events at a rapid clip, we could not rely on a relational database. Dataworkz uses a multi-modal persistence layer that includes Cassandra, relational, and graph databases, which are each really good at solving particular parts of the challenges.
We did not want to be worried about setting up Cassandra, maintaining it, and handling backups. We also did not want to provision large Cassandra clusters in the cloud with a minimum pre-defined capacity upfront. We explored cloud-native options and found that DataStax Astra DB met all our requirements for a column store. It was very easy to set up, with strong enterprise-grade security, fast queries, global availability, and scalability. In addition, we can restore a backup within 20 days, if needed. Best of all, we pay on a consumption-based model, which really helps a startup like ours control costs.
We’ve been happily using Astra DB for over a year now. We see our consumption growing as we sign up new customers.
How do you see the future for AI-driven decision-making?
Dataworkz is excited to democratize access to enterprise AI and data-driven decision-making tools. We aim to popularize the ability of business users to run data transformations in a very secure manner, without needing to go through the IT team for each new request or modification. Dataworkz gets rid of all that friction.
Today’s business users want to bring data from disparate systems, combine it for creating a holistic picture, and apply ML algorithms to make predictions without needing to jump through hoops using multiple tools. Dataworkz streamlines the process, whether you want to use disparate data for MLalgorithms or export into data visualization tools like Looker, Power BI, or Tableau with one click.
Many of the AI tools available today are like a black box. When you use off-the-shelf products, you don't even know what algorithm is being used. Dataworkz makes it all transparent. The ML catalog provides business users a list of available algorithms, such as for fuzzy logic, where you can use a Levenshtein distance or perhaps a Soundex phonetic algorithm to compare lists of names, depending on your use case and the kind of scoring model you need. We make it easy to execute the desired algorithm or enable users to bring in their own machine learning model.
How are you measuring the success of Dataworkz so far?
You can think of Dataworkz as a unified experience for building data-centric AI applications.
A recent customer of ours, a tech company based out of the San Francisco Bay Area, is testing our AI-driven data flows to create more complete, 360-degree views of their customers. Using the built-in ML algorithm they were able to disambiguate customer names sourced from third parties with internal data. The application uses a denormalized document model and they were able to convert a flat CSV file into a complex JSON document using Dataworkz’s no-code visual transformations in just a few clicks.
What would have taken months of work can now be done in a matter of minutes without the need for scarce technical resources. For us, that was a huge vindication of our approach that unifies “data + processing + AI” in a cohesive experience. It’s been a long time coming because in the data ecosystem, it can take four or five months to get introduced into an organization and make headway, especially with the comprehensive infosec reviews and security due diligence. But once you get in and demonstrate value, the flexibility offered by Dataworkz’s technology has enabled us to add additional use cases very quickly. We’ve been able to achieve this with our early adopters and that’s what propels us forward. We are really excited about what we are creating and what the future holds.
Read the full case study to learn more about why Dataworkz migrated to DataStax Astra DB.