Transforming Knowledge Discovery at Wikimedia
Wikimedia Deutschland launched an AI-powered knowledge project to enhance search and discovery across its vast community-driven repository of information on Wikidata. This initiative leverages DataStax's AI Platform, built with NVIDIA AI, to deliver cutting-edge vector search and knowledge graph capabilities. These advancements support Wikimedia's mission of providing millions of users worldwide with free, reliable, and contextually relevant knowledge. The collaboration addresses the need for scalable, AI-driven solutions to process and currently connects 115 million entities while maintaining the high standards of openness and accessibility central to Wikimedia’s principles.
30x faster query performance
90% reduction in development time
Support for 16 billion relationships
30x faster query performance
90% reduction in development time
Support for 16 billion relationships
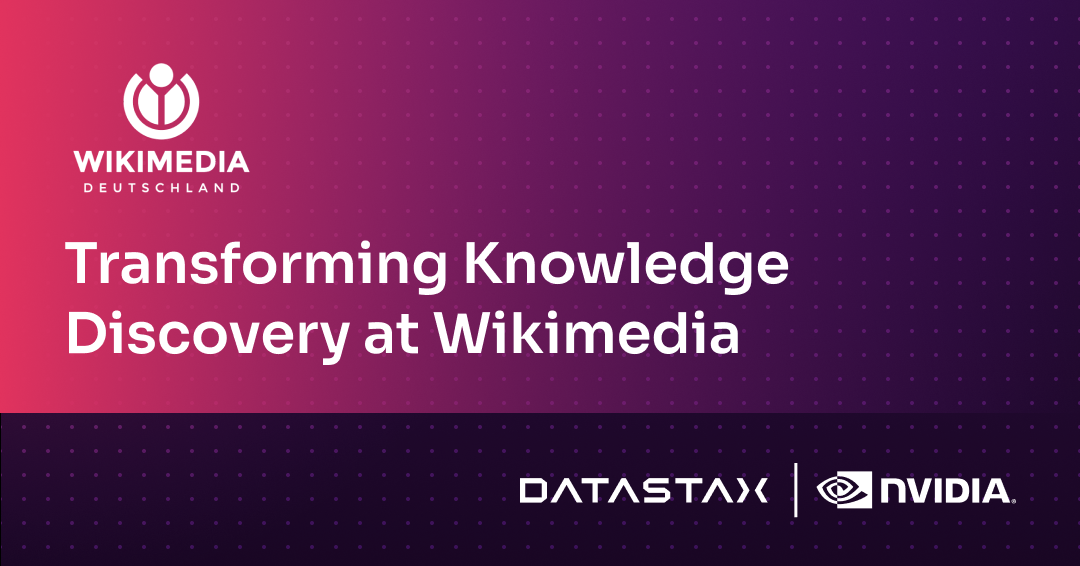
Challenge
As the global steward of free knowledge, Wikimedia faced challenges in delivering meaningful and precise search results to Wikidata users, primarily open-source developers. Not a small task given Wikidata’s rapidly growing, complex, yet high-quality dataset. Traditional keyword-based search methods couldn’t adequately interpret the context or relationships between entities, leading to suboptimal results for nuanced queries. Additionally, as the dataset expanded, Wikimedia needed to maintain low-latency search performance while managing the relationships between millions of entities in its knowledge graph. These challenges required a robust, AI-powered infrastructure supporting scalable and efficient workflows for user-facing search and backend knowledge curation.
“Our biggest challenge was ensuring that our search and discovery capabilities could keep pace with the growing complexity of our dataset. Traditional methods couldn’t capture the context and depth our users expect, and scaling those systems to support billions of relationships while maintaining performance was daunting,” said Lydia Pintscher, Portfolio Lead at Wikidata
Solution
To meet these demands, Wikimedia implemented DataStax's AI Platform, which integrates with NVIDIA NeMo Retriever, giving organizations a complete solution for all parts of the AI development and production lifecycle, from data ingestion to application deployment. Vector search was a key component of the solution, enabling Wikimedia to leverage vector embeddings for contextual understanding of search queries. This shift from keyword-based to semantic search enabled faster, more precise content retrieval, ensuring users could easily access the most relevant information.
The knowledge graph capabilities of DataStax’s AI platform enabled Wikimedia to model and explore complex relationships between entities across its dataset: this improved user navigation and the ability to derive meaningful insights from interconnected data. The integration of NeMo Retriever, included in NVIDIA AI Enterprise, brought GPU-accelerated performance to the platform, enabling Wikimedia to handle computationally intensive tasks such as generating embeddings and processing graph relationships in real time. The AI platform also allowed Wikimedia to scale seamlessly, ensuring reliability even during peak demand and simplified workflows, reducing the operational overhead of managing distributed AI systems.
Impact
The DataStax AI Platform has delivered measurable improvements, transforming how knowledge is accessed and managed:
- 30x faster query performance - Vector search capabilities dramatically improved the speed of information retrieval, enhancing the user experience for millions of daily users. With DataStax's AI Platform, Wikimedia was able to embed 10 million entities in just 3 days.
- 90% reduction in development time - AI-driven workflows drastically cut development cycles, accelerating feature deployment.
- Support for 16 billion relationships - The knowledge graph effectively models and manages billions of connections, enabling richer context and relevance in search results.
- Scalability across global workloads - GPU acceleration and distributed architecture provide seamless scaling to handle massive data volumes and concurrent queries.
- Operational efficiency - The unified platform reduced the complexity of integrating AI-driven tools, enabling Wikimedia to focus resources on innovation and content improvements.
"By leveraging DataStax's AI Platform, we’ve taken a giant leap in processing and delivering free knowledge. The combination of vector search and knowledge graph capabilities allows us to provide contextually rich and precise search results while ensuring scalability and efficiency to support our global community," noted Philippe Saadé, AI/ML Project Manager at Wikimedia Deutschland.
The collaboration between Wikimedia Deutschland and DataStax has redefined how free knowledge is accessed and managed globally. By addressing the limitations of traditional search methods and scaling to meet the demands of an ever-expanding dataset, this partnership has empowered Wikimedia to deliver a richer, more contextually relevant user experience via Wikidata. Leveraging state-of-the-art vector search and knowledge graph capabilities, supported by GPU-accelerated AI infrastructure, Wikimedia has enhanced its platform's performance and scalability and laid the foundation for future innovations in open knowledge sharing. This initiative underscores the transformative potential of AI in solving complex challenges and fulfilling Wikimedia’s mission of democratizing knowledge for all.