Cassandra Anti-Patterns: Queues and Queue-like Datasets | Datastax
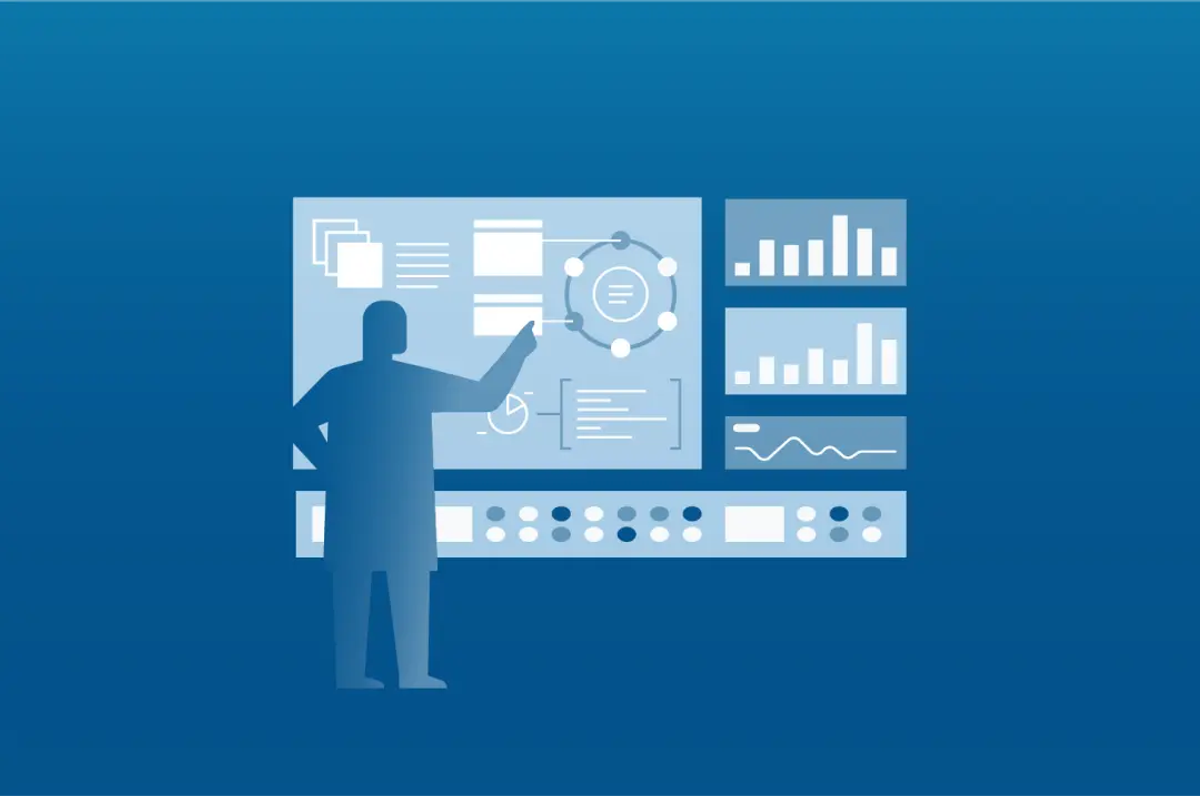
Deletes in Cassandra
Cassandra uses a log-structured storage engine. Because of this, deletes do not remove the rows and columns immediately and in-place. Instead, Cassandra writes a special marker, called a tombstone, indicating that a row, column, or range of columns was deleted. These tombstones are kept for at least the period of time defined by the gc_grace_seconds per-table setting. Only then a tombstone can be permanently discarded by compaction.
This scheme allows for very fast deletes (and writes in general), but it's not free: aside from the obvious RAM/disk overhead of tombstones, you might have to pay a certain price when reading data back if you haven't modeled your data well.
Specifically, tombstones will bite you if you do lots of deletes (especially column-level deletes) and later perform slice queries on rows with a lot of tombstones.
Symptoms of a wrong data model
To illustrate this scenario, let's consider the most extreme case - using Cassandra as a durable queue, a known anti-pattern, e.g.
CREATE TABLE queues (
name text,
enqueued_at timeuuid,
payload blob,
PRIMARY KEY (name, enqueued_at)
);
Having enqueued 10000 10-byte messages and then dequeued 9999 of them, one by one, let's peek at the last remaining message using cqlsh with TRACING ON:
SELECT enqueued_at, payload
FROM queues
WHERE name = 'queue-1'
LIMIT 1;
activity | source | elapsed
-------------------------------------------+-----------+--------
execute_cql3_query | 127.0.0.3 | 0
Parsing statement | 127.0.0.3 | 48
Peparing statement | 127.0.0.3 | 362
Message received from /127.0.0.3 | 127.0.0.1 | 42
Sending message to /127.0.0.1 | 127.0.0.3 | 718
Executing single-partition query on queues | 127.0.0.1 | 145
Acquiring sstable references | 127.0.0.1 | 158
Merging memtable contents | 127.0.0.1 | 189
Merging data from memtables and 0 sstables | 127.0.0.1 | 235
Read 1 live and 19998 tombstoned cells | 127.0.0.1 | 251102
Enqueuing response to /127.0.0.3 | 127.0.0.1 | 252976
Sending message to /127.0.0.3 | 127.0.0.1 | 253052
Message received from /127.0.0.1 | 127.0.0.3 | 324314
Processing response from /127.0.0.1 | 127.0.0.3 | 324535
Request complete | 127.0.0.3 | 324812
Now even though the whole row was still in memory, the request took more than 300 milliseconds (all the numbers are from a 3-node ccm cluster running on a 2012 MacBook Air).
Why did the query take so long to complete?
A slice query will keep reading columns until one of the following condition is met (assuming regular, non-reverse order):
- the specified limit of live columns has been read
- a column beyond the finish column has been read (if specified)
- all columns in the row have been read
In the previous scenario Cassandra had to read 9999 tombstones (and create 9999 DeletedColumn objects) before it could get to the only live entry. And all the collected tombstones 1) were consuming heap and 2) had to be serialised and sent back to the coordinator node along with the single live column.
For comparison, it took less than 1 millisecond for the same query to complete when no column-level tombstones were involved.
The queue example might be extreme, but you'll see the same behaviour when performing slice queries on any row with lots of deleted columns. Also, expiring columns, while more subtle, are going to have the same effect on slice queries once they expire and become tombstones.
Potential workarounds
If you are seeing this pattern (have to read past many deleted columns before getting to the live ones), chances are that you got your data model wrong and must fix it.
For example, consider partitioning data with heavy churn rate into separate rows and deleting the entire rows when you no longer need them. Alternatively, partition it into separate tables and truncate them when they aren't needed anymore.
In other words, if you use column-level deletes (or expiring columns) heavily and also need to perform slice queries over that data, try grouping columns with close 'expiration date' together and getting rid of them in a single move.
When you know where your live columns begin
Note that it's possible to improve on this hypothetical queue scenario. Specifically, when knowing what the last entry was, a consumer can specify the start column and thus somewhat mitigate the effect of tombstones by not having to either 1) start scanning at the beginning of the row and 2) collect and keep all the irrelevant tombstones in memory.
To show what I mean, let's modify the original example by using the previously consumed entry's key as the start column for the query, i.e.
SELECT enqueued_at, payload
FROM queues
WHERE name = 'queue-1'
AND enqueued_at > 9d1cb818-9d7a-11b6-96ba-60c5470cbf0e
LIMIT 1;
activity | source | elapsed
-------------------------------------------+-----------+--------
execute_cql3_query | 127.0.0.3 | 0
Parsing statement | 127.0.0.3 | 45
Peparing statement | 127.0.0.3 | 329
Sending message to /127.0.0.1 | 127.0.0.3 | 965
Message received from /127.0.0.3 | 127.0.0.1 | 34
Executing single-partition query on queues | 127.0.0.1 | 339
Acquiring sstable references | 127.0.0.1 | 355
Merging memtable contents | 127.0.0.1 | 461
Partition index lookup over for sstable 3 | 127.0.0.1 | 1122
Merging data from memtables and 1 sstables | 127.0.0.1 | 2268
Read 1 live and 0 tombstoned cells | 127.0.0.1 | 4404
Message received from /127.0.0.1 | 127.0.0.3 | 6109
Enqueuing response to /127.0.0.3 | 127.0.0.1 | 4492
Sending message to /127.0.0.3 | 127.0.0.1 | 4606
Processing response from /127.0.0.1 | 127.0.0.3 | 6608
Request complete | 127.0.0.3 | 6901
Despite reading from disk this time, the complete request took 7 milliseconds. Specifying a start column allowed to start scanning the row close to the actual live column and to skip collecting all the tombstones. The difference grows larger with size of the row increasing.
Summary
- Lots of deleted columns (also expiring columns) and slice queries don't play well together. If you observe this pattern in your cluster, you should correct your data model.
- If you know where your live data begins, hint Cassandra with a start column, to reduce the scan times and the amount of tombstones to collect.
- Do not use Cassandra to implement a durable queue.